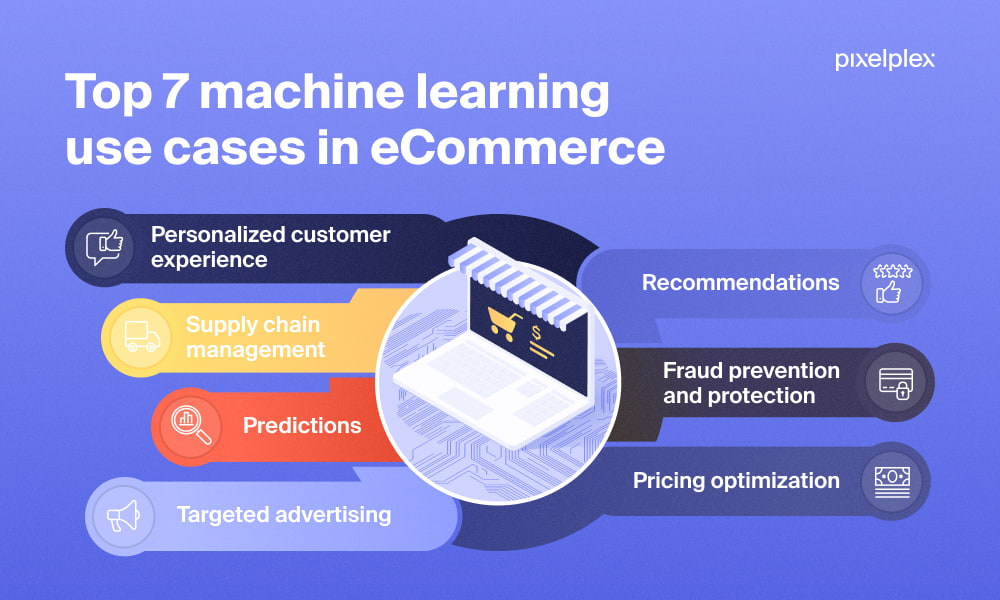
Introduction
Overview of Machine Learning
Machine learning has become a buzzword in the modern digital landscape, driving innovations across various sectors. At its core, machine learning enables computers to learn from data and improve their performance over time without being explicitly programmed. This technology empowers businesses to analyze vast amounts of information, uncover patterns, and make data-driven decisions. Imagine you’ve just purchased a new gadget online; next time you log in, your favorite e-commerce platform suggests accessories tailored just for you. That’s machine learning in action!
Some key components of machine learning include:
- Data Input: Machines learn from historical data to inform their predictions.
- Algorithms: These are mathematical formulas that help in making sense of the data.
- Continuous Improvement: As more data flows in, the models become even more refined.
Significance of E-commerce Optimization
In the competitive realm of e-commerce, optimization is vital for enhancing user experience and driving sales. Companies vying for consumer attention must adopt strategies that not only improve website performance but also cater to individual consumer preferences. E-commerce optimization involves refining various aspects, from product placement to inventory management, ensuring businesses can provide value consistently.
Consider this:
- Enhanced User Experience: A well-optimized site leads to faster load times and smoother navigation.
- Increased Conversion Rates: Personalized marketing strategies can effectively target customers, leading to higher sales.
- Stronger Customer Loyalty: When customers feel valued through curated experiences, they’re more likely to return.
At TECHFACK, we believe that understanding the impact of machine learning on e-commerce optimization is essential for businesses looking to stay ahead in this fast-paced digital landscape.
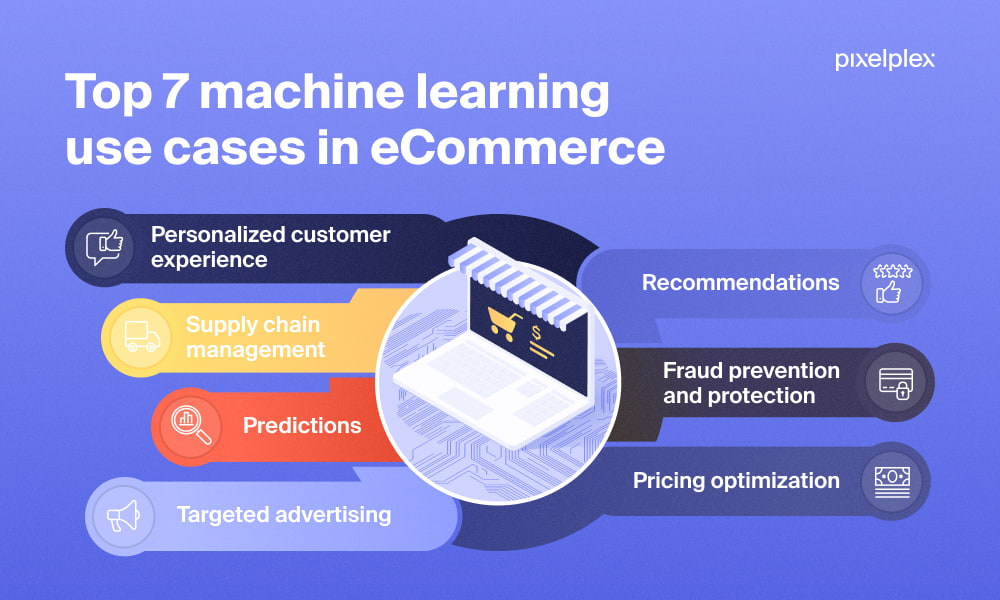
Understanding Machine Learning in E-commerce
Definition of Machine Learning in E-commerce
In the context of e-commerce, machine learning refers to the deployment of algorithms and statistical models that enable online retailers to analyze customer data and improve their offerings. This technology aids in predicting customer behavior, personalizing shopping experiences, and streamlining operations. For instance, when an online store recognizes that a user frequently buys running shoes, machine learning algorithms can suggest the latest gear or accessories based on their preferences. It transforms raw data into actionable insights, allowing businesses to make informed decisions that significantly enhance customer satisfaction.
Benefits of Utilizing Machine Learning
The adoption of machine learning in e-commerce comes with an array of benefits that can transform operations and elevate the customer experience. Imagine walking into a store where every product seems tailored to your taste; that’s the kind of experience machine learning can create online! Here are some tangible advantages:
- Enhanced Personalization: By analyzing past purchases and browsing behaviors, e-commerce platforms can create tailored marketing messages.
- Improved Customer Insights: Retailers can better understand their customers’ needs and preferences, leading to more effective product offerings.
- Efficient Inventory Management: Machine learning tools can predict stock levels, manage inventories effortlessly, and prevent overstocking or stockouts.
- Faster Decision-Making: Rapid data analysis allows businesses to react promptly to market changes, ensuring they remain competitive.
At TECHFACK, it’s clear that the integration of machine learning into e-commerce platforms is not just a trend; it’s a transformation that can substantially drive growth and elevate customer engagement.
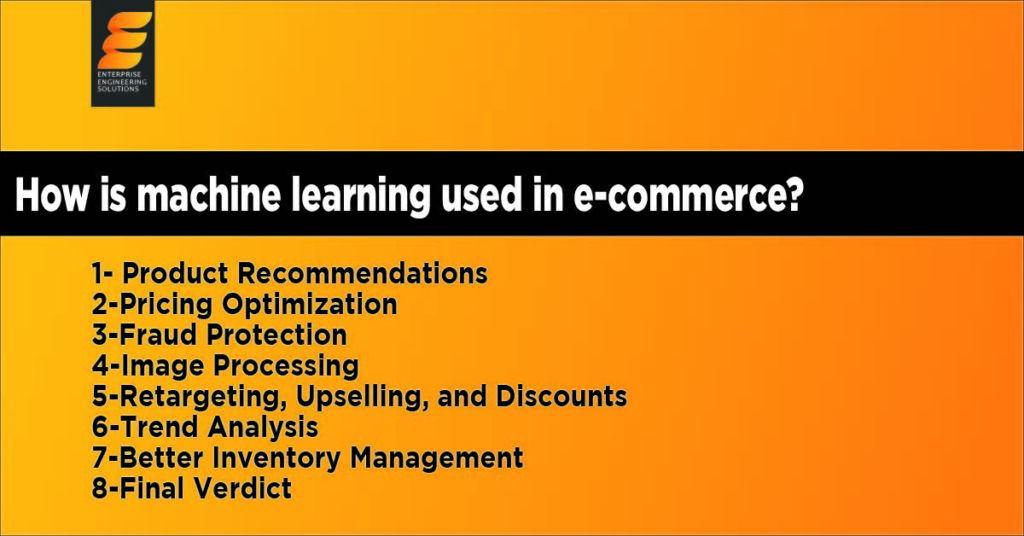
Impact of Machine Learning on E-commerce Optimization
Personalized Recommendations
One of the most significant impacts of machine learning on e-commerce optimization is the ability to deliver personalized recommendations. Picture browsing an online store, and every product on the homepage seems to resonate with your interests and previous purchases. That’s machine learning at work! By analyzing user behavior and preferences, retailers can provide tailored suggestions that enhance the shopping experience. This not only increases customer satisfaction but also drives sales.
For example:
- Collaborative Filtering: Analyzes users with similar tastes to recommend products.
- Content-Based Filtering: Suggests items based on the features of previously liked products.
Customer Segmentation
Another powerful aspect of machine learning in e-commerce is customer segmentation. It allows retailers to categorize customers into distinct groups based on buying habits, demographics, and preferences. This helps businesses tailor marketing strategies effectively. Imagine receiving exclusive offers that are perfect for your lifestyle, rather than generic promotions.
Key benefits include:
- Targeted Marketing Campaigns: Engaging specific segments with personalized content increases conversion rates.
- Resource Allocation: Helps businesses focus their resources on high-value customer groups.
Dynamic Pricing Strategies
Lastly, machine learning facilitates dynamic pricing strategies, enabling retailers to adjust prices in real time based on market trends, competitors’ prices, and demand fluctuations. This ensures that pricing remains competitive and profitable. Consider how clever algorithms analyze various factors, allowing e-commerce platforms to offer exclusive discounts when demand is low, while optimizing profits during high demand.
Elements of dynamic pricing include:
- Competitor Price Monitoring: Automatically adjusts prices to remain competitive.
- Predictive Analytics: Anticipates customer demand to smartly price products.
At TECHFACK, the transformative power of machine learning in these areas reveals how it can reshape e-commerce and significantly enhance customer engagement and profitability.
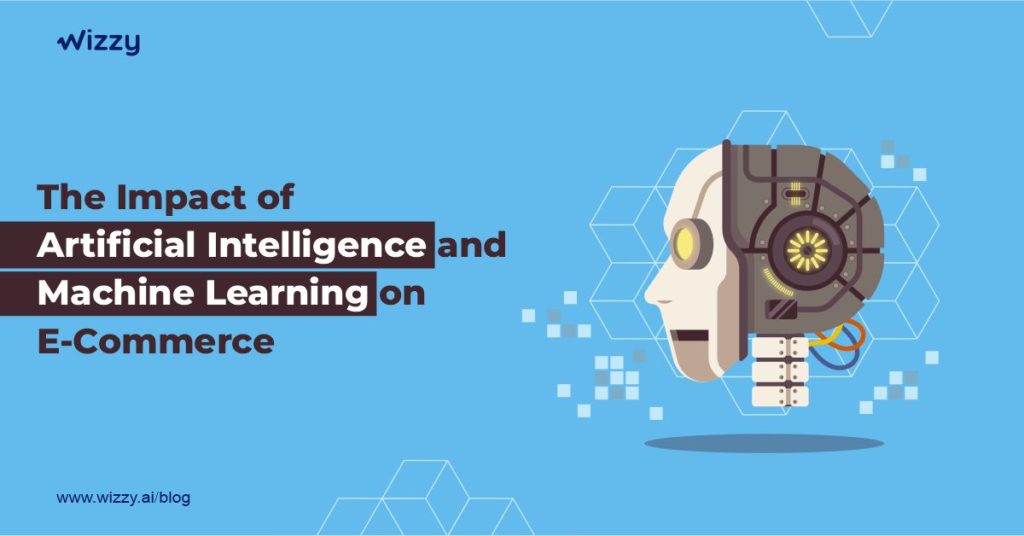
Implementing Machine Learning Techniques
Data Collection and Analysis
To harness the full potential of machine learning, the first step is efficient data collection and analysis. In e-commerce, data comes from various sources—customer interactions, transaction histories, web analytics, and social media. Imagine an online fashion retailer collecting data on what styles customers are browsing most frequently and which items tend to be abandoned in shopping carts. This data paves the way for actionable insights and helps tailor marketing strategies. Key elements to consider include:
- Quality of Data: Ensuring data is accurate and consistent.
- Diverse Data Sources: Combining different data types (quantitative and qualitative) for a holistic view.
A/B Testing for Optimization
Once the data is collected, the next phase is A/B testing, a powerful technique in machine learning implementation. This method entails creating two versions of an element within the e-commerce platform, such as product pages or email campaigns, to see which one performs better. For instance, a retailer can test different call-to-action buttons to determine which prompts more customer engagement. Important points to remember include:
- Clear Hypothesis: Define what you’re trying to improve and what success looks like.
- Statistical Significance: Ensure that the results are robust enough to inform future decisions.
Predictive Modeling for Inventory Management
Lastly, predictive modeling plays a critical role in inventory management. By utilizing historical data and trends, machine learning can forecast future product demand, enabling stores to maintain optimal stock levels. Think about a seasonal product, like winter coats; predictive modeling can help retailers prepare for demand spikes before the season hits. Key benefits include:
- Reduced Overstock Costs: Minimizing unsold inventory by accurately predicting demand.
- Improved Customer Satisfaction: Ensuring that popular items are available when customers want them.
At TECHFACK, it’s clear that implementing these machine learning techniques not only enhances operational efficiency but also significantly improves the overall customer experience in e-commerce.
Challenges and Future Trends
Data Privacy and Security Concerns
As the reliance on machine learning in e-commerce grows, so do the challenges, particularly concerning data privacy and security. With businesses collecting extensive customer information—from purchase histories to browsing habits—the risk of data breaches and misuse becomes a significant concern. Customers are becoming increasingly aware of how their data is used and are demanding transparency. For example, a friend of mine recently decided to stop shopping at a well-known retailer after learning about their data-sharing practices. Businesses must prioritize robust security measures and comply with regulations like GDPR to build customer trust. Important considerations include:
- Transparency: Clearly communicating how customer data will be used.
- Data Encryption: Safeguarding sensitive information against breaches.
Integration of AI and Machine Learning
Another challenge is the seamless integration of AI and machine learning technologies into existing systems. Retailers often find themselves juggling multiple platforms, making it difficult to create a cohesive strategy. It’s like trying to tune a band where every instrument is in a different key! To overcome this, companies should focus on a unified data strategy and invest in training AI and machine learning systems to work collaboratively across departments. Key strategies include:
- Cross-Department Collaboration: Ensuring marketing, sales, and IT teams work together.
- Incremental Integration: Gradually adopting machine learning processes to minimize disruptions.
Potential Innovations in E-commerce Optimization
Looking ahead, the potential for innovations in e-commerce optimization is exciting. With continuous advancements in technology, we can expect smarter algorithms that not only analyze data but also anticipate consumer trends. Imagine a future where stores utilize augmented reality to create immersive shopping experiences, allowing customers to “try on” products virtually. Other innovations on the horizon include:
- Voice Commerce: Enhancing shopping experiences through voice-activated technologies.
- Blockchain for Transparency: Utilizing blockchain technology to ensure product authenticity and traceability.
At TECHFACK, it’s evident that while challenges exist, the future of e-commerce optimization through machine learning will pave the way for remarkable innovations, addressing customer needs more effectively than ever.