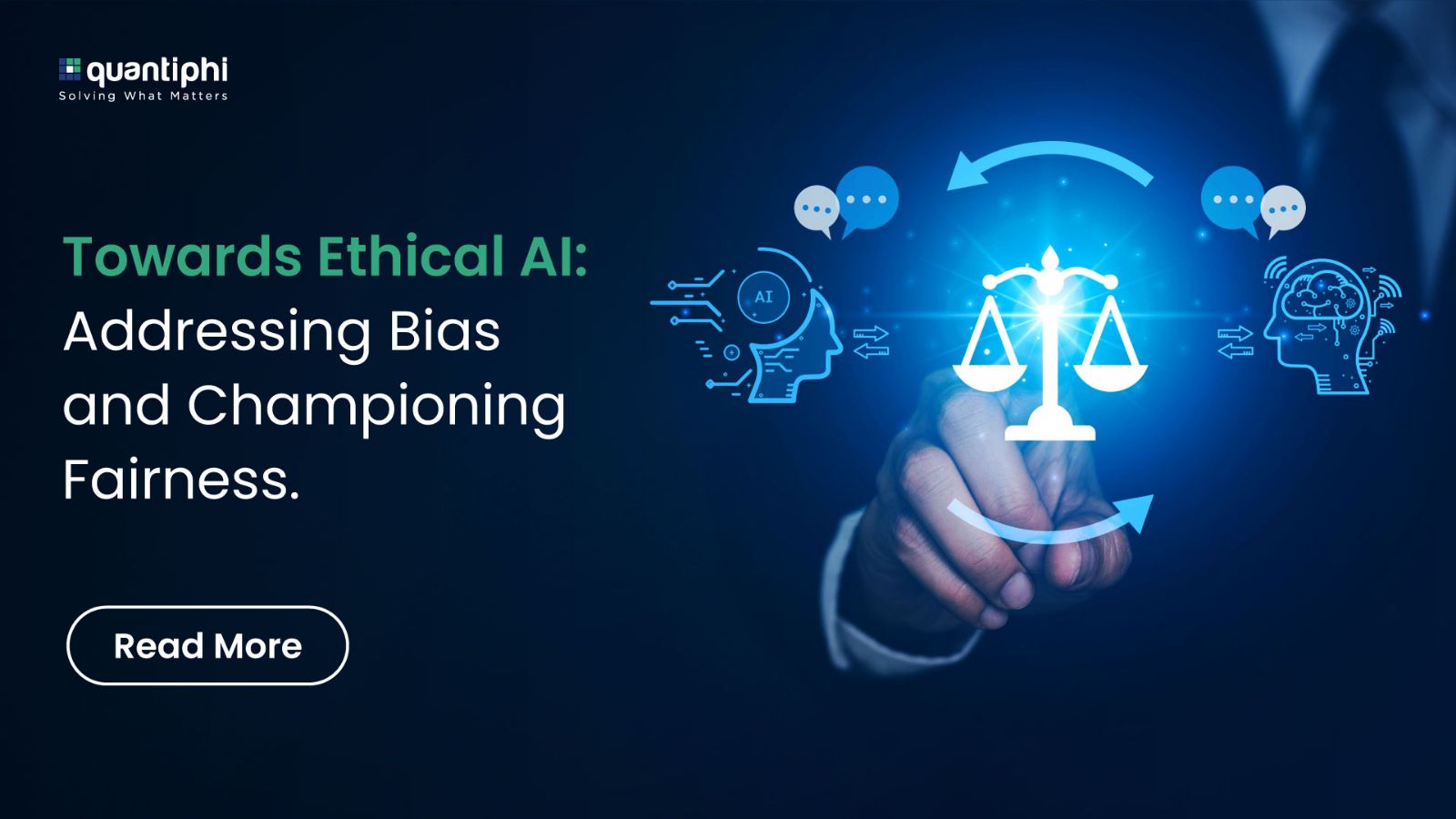
Introduction
Overview of Machine Learning
Machine Learning (ML) is a subset of artificial intelligence that enables systems to learn from data, improving their performance over time without explicit programming. This technology is rapidly transforming industries, from healthcare to finance, allowing businesses to make data-driven decisions with remarkable accuracy. For instance, imagine a medical diagnostic tool that analyzes patient data and suggests possible conditions; the enhancement in accuracy can save lives!
Importance of Ethical Considerations in Machine Learning
However, the rise of ML brings forth critical ethical considerations. Unchecked algorithms can perpetuate bias, leading to unfair treatment in various sectors. Don’t forget the recent discussions around biased algorithms in hiring processes; it’s a poignant reminder that:
- Transparency is essential
- Accountability must be enforced
- Fairness should be prioritized
At TECHFACK, we believe understanding these ethical implications is crucial as we navigate the exciting yet complex world of Machine Learning.
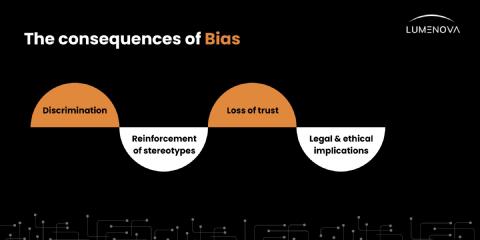
Understanding Bias in Machine Learning
Definition of Bias in Machine Learning
As we delve deeper into the ethics of Machine Learning, understanding bias becomes paramount. Bias in ML refers to systematic errors in algorithm outcomes that lead to unfair advantages or disadvantages for certain groups. This can skew decision-making processes, making it crucial for developers to recognize.
Types of Bias in Machine Learning
There are several common types of bias that can surface in machine learning models:
- Data Bias: This occurs when the training data is not representative of the real-world scenario. For example, if an image recognition system is trained primarily on images of one ethnicity, it may fail to recognize individuals from other backgrounds accurately.
- Algorithmic Bias: This is when the algorithm itself introduces bias in its decision-making processes. A classic case involved a credit scoring model that disadvantaged specific demographics, affecting their access to financial services.
- Representation Bias: This arises when certain groups are underrepresented in the dataset. For instance, if a dataset doesn’t include enough data from women, the resulting model may not perform well for female users.
Being aware of these biases helps ensure that ML evolves responsibly and ethically!
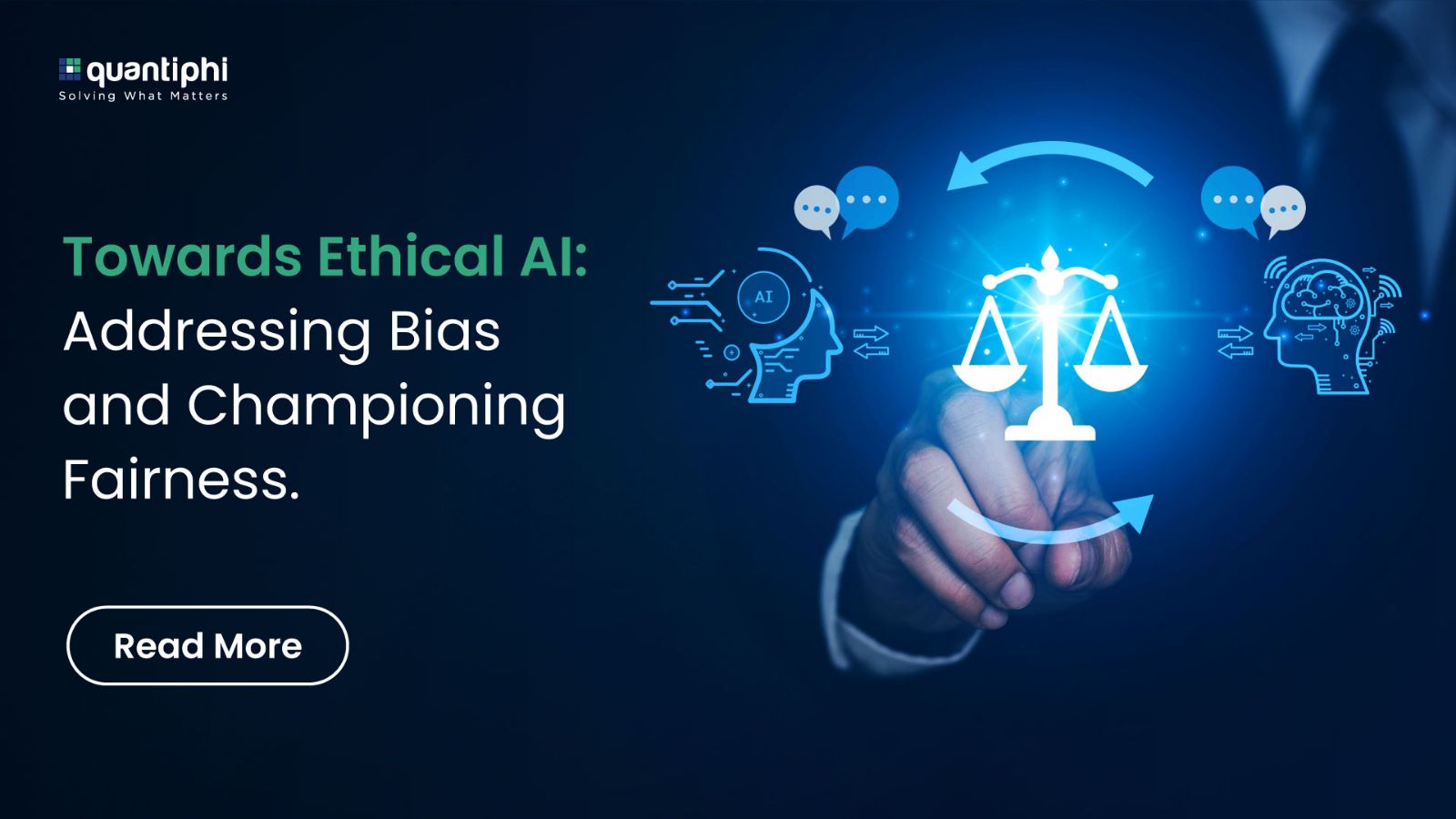
Fairness in Machine Learning
Understanding Fairness in ML Models
Building on our understanding of bias, let’s explore the concept of fairness in machine learning models. Fairness is about ensuring that the outcomes generated by these models do not favor any particular group over another. This is essential for maintaining trust and equity in ML applications, especially when they influence critical areas like hiring, healthcare, and law enforcement.
Fairness Definitions and Metrics
To assess fairness, researchers have developed several definitions and metrics, including:
- Equalized Odds: This metric evaluates whether different demographic groups have equal probabilities of receiving favorable predictions. For example, if two groups apply for a loan, they should have equal chances of approval, assuming similar financial profiles.
- Demographic Parity: Also known as statistical parity, this principle states that the decision rate should be the same across groups. In practice, if 70% of males are approved for a loan, the same percentage should apply to females.
- Calibration: Calibration refers to the accuracy of probability estimates across different groups. For instance, if a model predicts a 70% chance of loan approval, it should hold true across all demographics!
Fostering fairness in ML models isn’t just beneficial; it’s essential for creating a more equitable technological landscape!
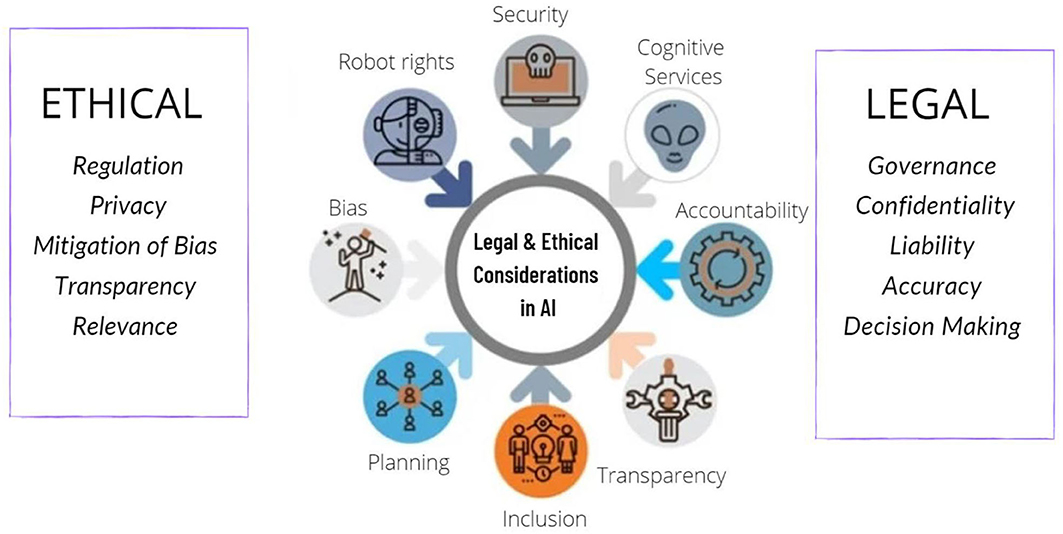
Ethical Challenges in Machine Learning
Impact of Biased Algorithms
Now that we’ve explored fairness, it’s essential to confront the ethical challenges posed by biased algorithms. These biases can lead to significant repercussions in real-world applications, ultimately affecting lives and communities.
Social and Ethical Implications of Unfair ML Models
Unfair ML models can manifest detrimental social and ethical implications, sparking widespread debates about the appropriateness of AI technology. For instance:
- Discrimination: Biased algorithms can unfairly disadvantage certain demographic groups, leading to systematic inequalities. Imagine a hiring algorithm that favors male applications over equally qualified female candidates—this not only perpetuates gender bias but also hinders workplace diversity.
- Privacy Concerns: Many ML models rely on personal data to function. Mismanagement of this data can lead to violations of privacy rights, raising eyebrows in an era where data breaches are commonplace.
- Lack of Transparency and Accountability: Many ML systems operate as “black boxes,” making it challenging to understand their decision-making processes. This opacity can further complicate accountability when policies need enforcement.
Addressing these ethical dilemmas is vital for creating a trustworthy and equitable tech environment where innovation flourishes!
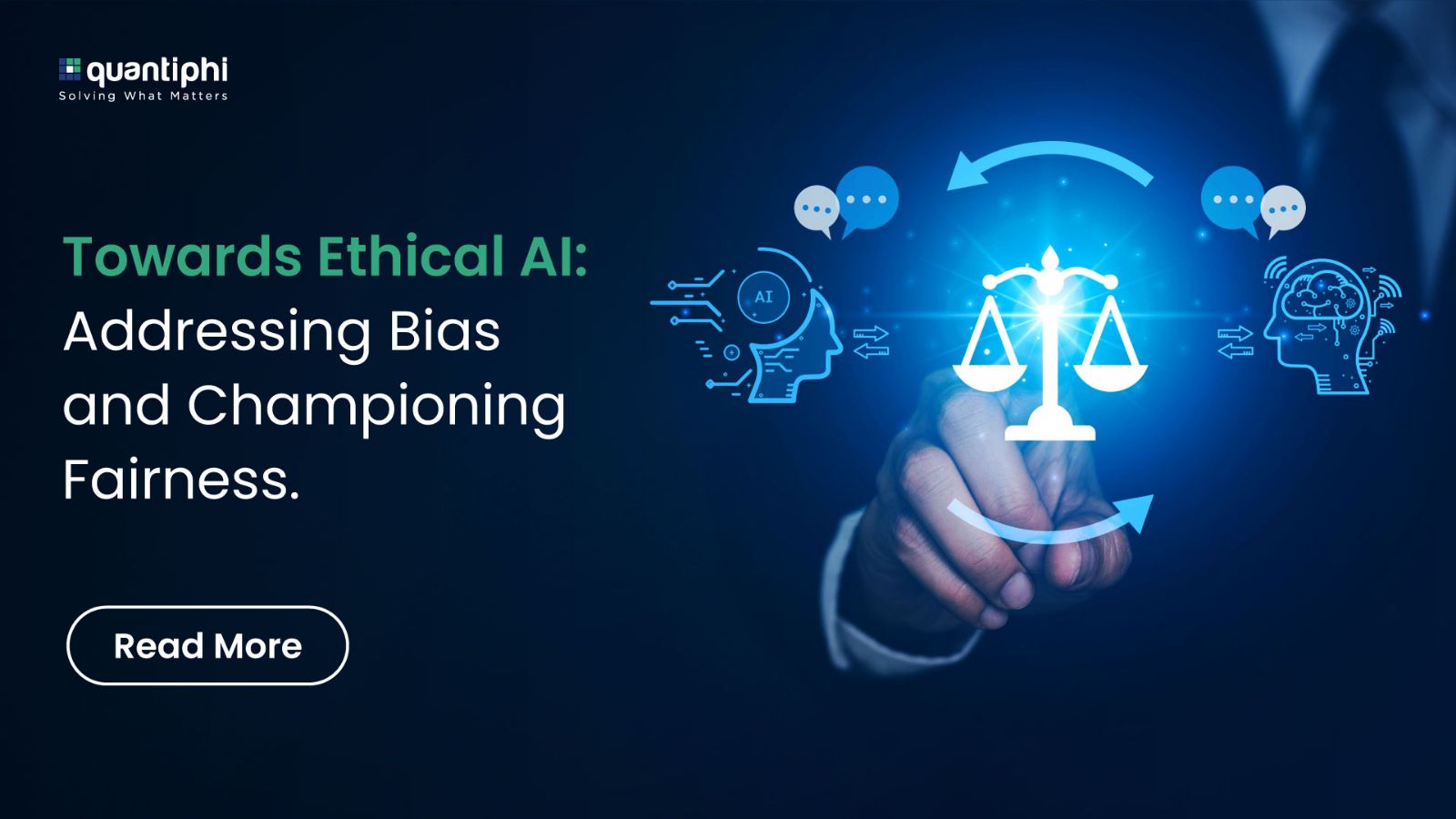
Addressing Bias and Ensuring Fairness
Bias Mitigation Techniques
Having tackled the ethical challenges of machine learning, let’s now focus on addressing bias and ensuring fairness. There are several effective bias mitigation techniques that can help create more equitable models.
Fair Representation Techniques
One approach is utilizing fair representation techniques, which restructure the input data to neutralize bias. For example, oversampling underrepresented groups can ensure that a minority has a voice in the data.
Post-processing Equalization
Post-processing equalization adjusts the output of algorithms after learning has occurred. This method allows for corrections without needing to redesign the original model—imagine recalibrating the predictions to achieve fairer outcomes!
Implementing Fairness in ML Models
When implementing fairness measures in ML models, integrating fairness-aware machine learning algorithms is key. These algorithms explicitly factor fairness into their objective functions, making fairness a priority rather than an afterthought.
Fairness-aware Machine Learning Algorithms
Last but not least, enhancing interpretability and explainability of these algorithms helps demystify their decision-making processes. Users can understand why decisions were made, fostering trust and accountability in machine learning systems! By addressing bias proactively, we shape a future where technology serves everyone equitably.

Regulatory Frameworks and Guidelines
Overview of AI Ethics Guidelines
As we’ve seen, ensuring fairness in machine learning is vital, and regulatory frameworks play a pivotal role in guiding this journey. Recognizing the impacts of AI technologies, various guidelines have emerged to shape ethical practices.
Ethical AI Principles from Global Organizations
Global organizations have established ethical principles to steer AI development. Common themes include:
- Transparency: Encouraging open communication about how AI systems function.
- Accountability: Ensuring responsible parties can be identified for AI decisions.
IEEE Global Initiative for Ethical Considerations in AI and Autonomous Systems
The IEEE Global Initiative focuses on establishing ethical standards specifically for AI and autonomous systems. They advocate for human-centric design, prioritizing user welfare and societal good, which resonates well with AI practitioners aiming to make a positive impact.
EU Ethics Guidelines for Trustworthy AI
Additionally, the EU’s Ethics Guidelines for Trustworthy AI emphasize key requirements, including accountability, privacy, and the ability to challenge decisions made by AI systems. These guidelines not only promote fairness but also ensure a comprehensive approach for integrating ethical considerations into AI practices.
With a solid foundation of ethical guidelines, we can look forward to a future where technology uplifts society responsibly!

Case Studies and Examples
Real-world Examples of Bias in Machine Learning
As we navigate the complex landscape of AI ethics, examining real-world examples of bias in machine learning becomes crucial. For instance, a well-known case occurred with a hiring algorithm deployed by a major tech company. This system inadvertently favored male candidates due to historical hiring patterns in the dataset. The result? A significant underrepresentation of women in technical roles, highlighting the urgent need for bias mitigation.
Ethical Dilemmas in AI: Case Studies
Moreover, ethical dilemmas arise when deploying AI in sensitive areas. In a notable case involving facial recognition technology, a study found that the algorithm was misidentifying individuals of certain ethnic backgrounds at disproportionately higher rates. This not only raised alarm bells about fairness but also sparked discussions on the accountability of tech companies.
Through these case studies, we see the profound impact of ethical considerations in machine learning and the ongoing need for vigilance and proactive solutions. By learning from these instances, we can strive for a more fair and responsible AI landscape!

Conclusion and Future Directions
Summary of Key Takeaways
As we wrap up our exploration into the ethics of machine learning, several key takeaways emerge. First, bias and fairness are critical considerations in developing ML systems, directly impacting trust and equity. Real-world examples have shown the pitfalls of biased algorithms, underlining the importance of proactive measures.
- Awareness of Bias: Understanding the different types of bias, such as data and algorithmic bias, is essential.
- Frameworks for Fairness: Regulatory guidelines exist to guide ethical practices in AI.
Future Directions in Ethical Machine Learning Research
Looking ahead, future directions in ethical machine learning research may involve deeper dives into interpretability and enhanced fairness-aware algorithms. Researchers may explore advanced techniques that blend fairness and performance, finding innovative ways to ensure ethical outcomes. Engaging a diverse range of stakeholders in the development processes will also be vital in crafting solutions that truly serve everyone.
It’s an exciting and critical time to prioritize these ethical considerations, shaping a future where technology can benefit all of humanity!